
If you do not play the lottery, you won't gain or lose anything. The Risk Tolerance 'R' has a very intuitive interpretation that makes its assessment very easy.Ĭonsider a decision situation where you can choose to play a lottery or not to play a lottery. How can the Risk Tolerance (R) be determined? Approximate method So, we can whimsically use this utility function, but of course, there is no scientific ground that proves that human attitude perfectly follows this utility function. Then notice that the utility for any negative x (being in debt) is negative, which makes perfect sense too. That makes sense as someone who does not get anything, his utility is 0, right?. The utility of zero in this equation, U(0), is equal to 0.

If you look into this utility function ( ), you will notice that, as x increases, U(x) approaches 1, which means the highest utility.

It is just an idea that, as such function is concave, it can be used to model the behavior of a risk-averse decision-maker. The human brain and behavior are more complex than such a modeling function. Well, obviously, this function was not derived. From where this utility function comes from The exponential utility function is mainly used to measure the utility of monetary gain where there is a chance of losing money. If you are less tolerant of risk, you would assess a smaller R and have a more curved utility function. Thus, if you are less risk-averse, (you can tolerate more risk), you would assess a greater value for R to obtain a flatter utility function. When your risk tolerance is infinite, the above function becomes a straight line equation. Greater values of R (Risk Tolerance) make the exponential utility function flatter, while smaller values make it more concave or more risk-averse. In the above chart, we used the Risk Tolerance value (R) = 1000. In such a utility function, R, the Risk tolerance parameter determines how concave the utility function is, which in turn reflects how risk-averse the decision-maker is. This utility function is concave, and so it can be used to model risk aversion. If you represent real-world money gain on the X-axis and your level of satisfaction on the Y-axis (in terms of 0 to 1, where 0 means no satisfaction and 1 means the highest satisfaction), then the Exponential Utility function will look like this:
#Power utility function inverse software
Decision Tree Software can calculate that parameter based on the Minimum and Maximum possible values in the decision context, which is collected from the user. "a" and "b" are essentially scaling parameters. x is the real-world value and u(x) is the utility value or perceived value (the value of an outcome in utils). Formally, the exponential utility is given by: This utility function is mainly used for mapping real-world Monetary gain to perceived value. In economics and finance, exponential utility refers to a specific form of the Utility Function. But mean-variance analysis or CAPM generally are hardly used in practice, but rather as conceptual tools, I'd say.Exponential Utility Function What is the Exponential Utility Function? Or are the issues I cited about the quadratic just negligible in practice? Not aware of a clean portfolio optimisation theory under more realistic assumptions. For example, a mean-variance investor could refuse a gift of a limited liability asset, if it's volatile enough. That's complicated.Īre there no other simple, more realistic functional forms for utility that would still lead to a reasonably clean portfolio optimization theory? Impose joint conditions on both the expected utility function and the distribution of $W$.
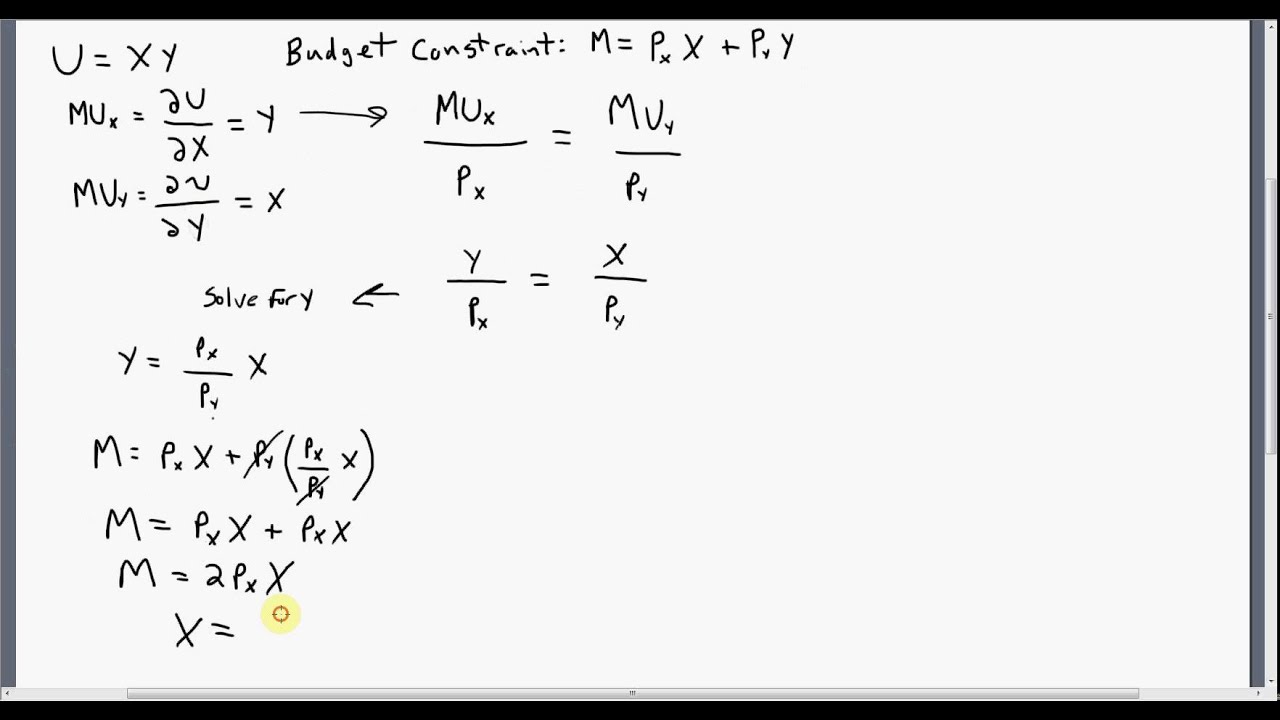
Those can have a restricted domain (ie limited liability, though then also limited upside, if I'm not mistaken). It can be shown that one can express the above as a function of only $\mu, \sigma$ for elliptical distributions, no matter what $U$ is chosen. Impose conditions on the returns, ie the distribution of $W$. That is the idea with quadratic utility (which, as you point out, has many problems). Impose conditions on the utility function: Take an expected utility function $U$ whose higher derivatives (above second derivative) vanish. Now, to turn this into a mean-variance utility (ie only a function of $\mu, \sigma$), there are 3 ways: You can express the expected utility using its Taylor approximation around the expected wealth, with $\mu = E, \Delta = W - \mu$ (note that $E=0$): This is an entirely different approach than expected utility maximisation. The capital asset pricing model (CAPM) is based on mean-variance utility investors choose their portfolio based only on its mean and variance.
